-
Transactional advisory services
Find out more about the transactional advisory services of Grant Thornton Financial Advisory Services
-
Valuations
Find out more about the valuations services of Grant Thornton Financial Advisory Services
-
Mergers and acquisitions
Find out more about the merger and acquisition services of Grant Thornton Financial Advisory Services
-
Forensic and investigation services
Find out more about the forensic and investigation services of Grant Thornton Financial Advisory Services
-
Recovery & reorganisation
Find out more about the Recovery & reorganisation services of Grant Thornton Financial Advisory Services
-
Business risk services
Find out more about the business risk services of Grant Thornton Financial Advisory Services
-
Business consulting
Find out more about the business consulting services of Grant Thornton Financial Advisory Services
-
Capital market
Capital market
-
Corporate and business tax
Find out more about our corporate and business tax services.
-
Direct international tax
Find out more about our direct international tax services.
-
Global mobility services
Find out more about our global mobility services.
-
Indirect international tax
Find out more about our indirect international tax services.
-
Transfer pricing
Find out more about our transfer pricing services.
-
Litigation
Our lawyers and accountants can manage all defense measures provided not only by the Italian law, but also by EU regulations and conventions
-
Family business
Find out more about our Family business services.
-
Legal
The client can be assisted in every need and with the same care both on important operations or disputes and on simple matters
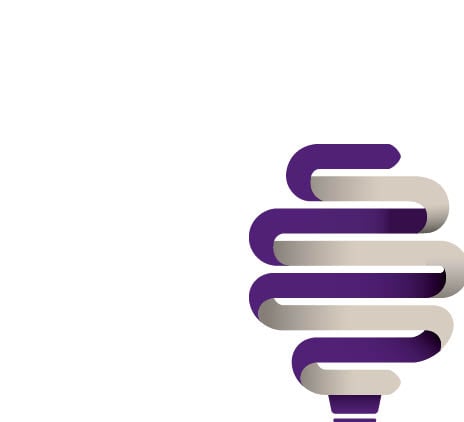
-
Back office outsourcing
Find out more about our Back office outsourcing services
-
Business process outsourcing
Find out more about our business process outsourcing services.
-
Compilation of financial statements
Find out more about our compilation of financial statements services.
-
Tax compliance
Find out more about our tax compliance services.
-
Electronic invoicing
Find out more about our electronic invoicing services
-
Electronic storage
Electronic storage is an archiving procedure that guarantees the legal validity of a digitally stored electronic document
-
Revaluation of corporate assets
Find out your civil and fiscal revaluation of tangible, intangible and financial assets
-
Human resources consulting
Find out more about our human resources consulting services.
-
Payroll
Find out more about our payroll services.
-
HR News
HR News the monthly information newsletter by Grant Thornton HR
-
Cybersecurity
GT Digital helps clients structure information security management internal functions, also through partially or totally outsourced functions
-
Agile and Programme Management
GT Digital provides support in the adoption and implementation of different portfolio management
-
Robotic Process Automation
Our “BOT Farm” can rely on digital workers able to help clients in routine activities, allowing employees to deal with more added-value activities
-
Data strategy and management
GT Digital can support clients in seizing the opportunities offered by Big Data, from the definition of strategies to the implementation of systems
-
Enterprise Resource Planning
We support clients in selecting the most appropriate ERP System according to their specific needs, helping them also understand licensing models
-
IT strategy
GT Digital supports clients in making strategic choices, identifying innovation opportunities, comparing themselves with competitors
-
IT service management
We can support with software selection and with the implementation of dedicated tools for the management of ICT processes
-
DORA and NIS 2
The entry into force of the DORA Regulation and NIS2 represents a major step towards the creation of a harmonised regulatory framework
Discrimination occurs when a person is denied an opportunity or a right due to a certain opinion based on groundless and inappropriate reasons. Sometimes, artificial intelligence systems are not impartial and show prejudices that result in discriminatory algorithm outputs against an individual or a group of individuals due to their race, gender, age, etc.
From a scientific perspective, this phenomenon is known as “bias”, i.e., a distortion caused by prejudice. This does certainly not concern only algorithms, but also human minds.
Humans have cognitive bias, i.e., systematic distortions of judgment that, substantially, derive from two different sources: the first one is biological, while the second one is the result of the cultural and social context in which an individual grows up and lives.
We can affirm that the first source is “infrastructural” and the second one is “informational”.
For example, the order in which options are presented conditions human decisions: the human brain “prefers” some things over others because they are presented in a certain position compared to others. An example is the way products are presented on supermarket shelves or the way movies are presented on platforms. This is “infrastructural” bias.
Typical “informational” bias is gender bias. If we are used to see men at top positions, we could be inclined to incorrectly believe that women cannot reach leadership positions.
Artificial intelligence systems are potentially subject to the same distortions or bias that condition humans.
An artificial intelligence system being used to suggest the most suitable applicant for a vacancy could provide unappropriated suggestions due to infrastructural reasons related to the algorithm, which could have some defects causing a certain characteristic to be under- or overestimated. Otherwise, it could provide a wrong or discriminatory suggestion due to informational reasons, i.e., reasons related to the data used to “train” the system.
In fact, data could present distortions just like the environment in which humans grow up: in fact, they are a product of such environment. Hence, the expression “garbage in garbage out”: if data are of poor quality, algorithm predictions will be of poor quality, too, and will generate errors or distortions.
A typical area in which bias can be found is that of equality between men and women or between people of different races or religions, because our economic and cultural system has been based for millenniums on discriminatory behaviours, which are often supported by legal systems.
If female applicants in a certain company have always received a lower remuneration than male ones, an algorithm used by an artificial intelligence system that was trained on those data will be inclined to propose women a lower average remuneration. This kind of distortion is called historical bias and, in many cases, it is the result of a social inequality in training data.
The emerging Artificial Intelligence Act (please refer to Carlo Giuseppe Saronni’s article in this TopHic issue) should also deal with this kind of bias.
In fact, art. 29 (a) of the text proposed by the Parliament provides for an impact assessment on fundamental rights, based on which the system user should make an assessment on high-risk systems aimed to identify potential breaches of fundamental rights, including the right to non-discrimination.
As said, bias can also depend on a poor representativeness of data.
In general, the more the data received by an artificial intelligence system on a certain area or matter or on a certain population, the more solid and reliable its prediction and capacity to represent it properly.
Consider, for example, racial discrimination: if few data are available for a certain race, the system prediction on that race will not be fully reliable since the system is trained on few information.
Then, if characteristics (e.g., low income) are constant for that race, the system will associate a certain race with a low income also in opposite circumstances, thus damaging persons belonging to that group.
To solve the low representativeness of data, the regulation should provide under art. 10 (3), that “data must be representative” of the population which the algorithm has effect on.
The rationale behind the regulation is commendable.
However, it is difficult to concretely comply with this provision.
In fact, data available to the algorithm will always depend on the reference population up to that moment and, therefore, it will sometimes be difficult to avoid some cases of misrepresentation.
Lastly, some kinds of bias depend on the type of data used.
There are some human characteristics that can hardly be represented.
Intelligence, moral sense, empathy, the ability to conciliate people are aspects that can hardly be represented by measurable data and, subsequently, evaluated, for example, by a personnel recruitment algorithm.
In this case, again, the output provided by the machine could be discriminatory or non-efficient for the user, since it could lead to the selection of a less deserving applicant only because fundamental qualities are not captured by data and therefore are not considered.
One last example.
Intelligence is a complex of mental faculties that distinguishes humans from animals.
IQ can be measured. However, IQ regards a very limited aspect of human intelligence, i.e., logical abilities (elementary inferences that involve short-term memory) and spatial visualization (most of all, rotation and pattern recognition).
These abilities, either innate or trained, certainly favour those professions that are more based on spatial reasoning, such as mathematicians and physicists, who have a higher preparation in geometry. These individuals will be favoured by an artificial intelligence algorithm that measures human intelligence based on IQ.
In fact, even if the machine is very sophisticated, it needs an element to be measurable, in order to give it a value.
In this sense, IQ is a measurable, established, and privileged parameter to quantify intelligence.
Nonetheless, this parameter cannot properly capture the whole dimension that it aims to measure, i.e., intelligence, since measurable data could weight more than other important – but non easily measurable – personal qualities.
Therefore, the evaluation of intelligence based on IQ represents a synthetic but not exhaustive solution to represent the characteristics of an applicant, because the system could exclude deserving individuals due to the impossibility for its impossibility to process hardly measurable aspects of intelligence.
Clearly, these are very sensitive issues.
Data scientists cannot be left alone in considering these problems but should rather be supported by jurists and philosophers who can help them identify the possible bias in the artificial intelligence system and the best technologies to mitigate them.
Luckily, large companies are recruiting these professional figures, who should help data scientists organize their work.